March 8, 2021
People or Machine: The Emerging Gap Between Fully Automated Risk Selection and Human Intervention in Cyber Insurance
John Merchant is Managing Director, Cyber and Technology Practice – North America for Optio Insurance Services, a global MGA specializing in over 13 classes of business. He is responsible for overseeing business development, digital distribution strategy, product development and vendor management. Prior to joining Optio, John was the Insurance Practice Advisory Leader and Cyence Risk Analytics and was responsible for the planning and execution of strategic customer initiatives. Prior to joining Cyence, John spent over 10 years in the insurance industry, primarily focused on cyber product development, strategy and underwriting. John has held positions at Nationwide, AIG and The Hartford. Before joining the insurance industry, he held various strategic sales roles in the technology sector. John holds a BA in Political Science from the University of Connecticut.
I underwrite from my gut. A statement no senior executive wants to hear from their lead cyber underwriter, or any underwriter for that matter. I can attest to hearing that statement, or something similar on occasion though. Although an extreme example, it’s hard to deny that a certain degree of empirical reasoning goes into many cyber underwriting decisions, as it should. To what degree empirical data, or gut instinct, influences underwriting decisions could be the difference between profitability and being in the red. Sometimes those decisions, although appearing to be gut instinct, are based on sound experience and are a crucial part of the decision-making process.
With any extreme approach an opposite must exist. In the case of cyber underwriting, a purely data-driven approach without any human underwriter involved doesn’t exist just yet (excluding cyber endorsements and other slot-rated products for very small entities). However, the industry has begun to more fully embrace a risk selection approach heavily influenced by externally collected and curated data. Investors have too. Five years ago, there were no insurtech Cyber MGAs. Now there are over a half dozen and counting, and funding appears plentiful.
For this blog post I’d like to pose a question. Which approach is better? I’d argue a cogent case can be made for both, especially given the current market conditions. There’s no denying that data and analytics are here to stay. Predictive modeling and analytics have changed the landscape of several industries. For me, an industry most profoundly changed is professional baseball. Other industries have been changed materially as well, but baseball is more interesting to note than financial services. Apologies to all the quants out there.
The arc undertaken by baseball is reminiscent of the arc being taken by the cyber insurance market. A very old industry, set in its ways, being unexpectedly and somewhat begrudgingly forced to adopt the use of data to make decisions.
This shift from a game dominated by gut decisions, feeling and how a player looked to one dominated by predictive analytics was seismic. Who knew game decisions and multimillion dollar contracts would be made by acting almost solely on OPS, ISO, WHIP or wOBA. All acronyms I’d be happy to debate on the next sabermetrics Zoom. However, have these analytics proven to be superior or is the human element (meaning decision making by informed instinct) a necessary or even superior method in some cases?
Below are mini, non-lawyerly cases for a machine approach to underwriting vs. a human approach. I purposely avoided making cases against each as they are implied.
The Case for Machines
Were already there. Auto, home, BOPs…all underwritten by algorithms gathering internal and external data to predict profitability at the policy level. These are standardized products of course, but human touch is no long part of the process. This allows for lower acquisition costs and the ability to grow at scale. Cyber however, is anything but commoditized. I could argue the industry has tried a little too hard to commoditize a highly complex product, but that’s fodder for another blog. That thought aside, the introduction of externally collected and curated data to drive the underwriting decisions has exploded.
Data can be collected and analyzed at scale, providing threat intelligence which is simply impossible to ascertain through the traditional underwriting process. There is no way, at least from what I’ve seen, that an underwriter can quickly find out the number of publicly facing IP addresses, proper or improper configuration of the Sender Policy Framework (SPF), whether traffic to and from a website is encrypted and if that encryption is strong or weak, and finally, if any RDP ports are externally visible and therefore prone to exploitation by cyber extortionists. Underwriters could ask on an application, but good luck with that approach.
Our machine friends can also provide portfolio level intelligence to ascertain potential points of accumulation. With these points of accumulation identified, they can assist with building disaster scenarios, which up until recently were built by adding ones exposed limits together. I’m sure many of my colleagues on the underwriting side recall the days when accumulation management meant asking what cloud providers a company used and then physically adding them to an excel spreadsheet. A tedious task, I assure you.
Are these models perfect. No, far from it. They’re all wrong to some degree but are certainly better than knowing nothing. To grossly oversimplify, I’d rather be told there’s an 80% chance it may rain this weekend and prepare appropriately than plan a beach day on what turns out to be a wash out.
To conclude the case for the machines, this data can be continually collected, and models trained to get better and more accurate. Economies of scale kick in and this approach becomes less and less expensive, in theory.
The Case for People
You can’t have a beer with a machine. Twenty years ago, that may have been a valid case. Kidding aside (sort of), models and the data and analytics that power them are only as good as their creators, who are people. Not underwriters, but people, nonetheless. Where underwriters come in is in the interpretation of the data. As pointed out, all models are wrong to some degree. That degree is ascertained by highly trained underwriters with years of experience in the market. Plus, the human brain is still the best decisioning engine ever created.
There are also several risk factors which can’t be gleaned from an external scan or continuous monitoring of a network. These include how (or if) companies train their employees, the experience level of their management and security team, the health of the industry class, the overall adoption of a security mindset, and their plans in the event of a cyber-attack. None of these data points can be gathered externally, but any claims rep or breach coach will tell you they’re directly correlated with losses.
To conclude the case for people, this is still a relationship business. Something I hope will not go away. Trust is arguably the most important asset an underwriter can have and is only gained through long-term relationship building.
The Case for Both
It doesn’t take a rocket scientist (or an algorithm) to predict where I was going with this blog post. However, its not quite that simple. It’s too easy to say that underwriters should simply merge their approach with that of a data only approach, attaining some level of cyber zen. This is where the real work comes in, and where I have some direct experience.
Having worked at a predictive analytics start-up prior to joining Optio, I saw first-hand how powerful these models could be. However, two common themes among my clients also struck me.
One was how much work the customer, in this case insurers and reinsurers, had to put in to glean real value from the model. There’s heavy lifting to be done on the part of the customer to make these solutions work. Often this additional work wasn’t anticipating by the customer, creating agitation and unwelcome extra work.
Second, and more importantly in my opinion, was the analytics telling an underwriter something they didn’t want to hear. Prior to 2019, incurred losses were in the 40% range, with actuals much lower. Unexpectedly seeing a profitable portfolio showing a modeled loss ratio 20-30 points higher instills almost immediate distrust in the analytics. Also, competing models may show materially different loss figures, adding to this distrust. On an individual risk selection level, for an underwriter to be told that a company they’ve been insuring for several years, without any notices, is suddenly a bad risk raises questions, as they should. It’s an underwriter’s job to question.
These issues can lead underwriters to use the good news and filter out the bad, effectively gaming the system. On the flip side, going solely by the numbers may have led to no growth and, not to be crass, but no bonus or job. Last time I checked those were important.
The case for both is a challenge to the cyber underwriting industry, both old guard and new arrivals. On one side a challenge to recognize that machine-learning and AI can be a tremendous competitive advantage but utilizing them is a major undertaking and some level of trust is required. On the other side, a challenge to recognize that pure data-driven underwriting with no, or very limited underwriter oversight can lead to losses at scale and increased distrust of technologies that are vital to a profitable cyber insurance market.
Thank you, and I welcome your feedback and opinions.
News Type
PLUS Blog
Business Line
Cyber Liability
Topic
Professional Liability (PL) Insurance
Contribute to
PLUS Blog
Contribute your thoughts to the PLUS Membership consisting of 45,000+ Professional Liability Practitioners.
Related Podcasts
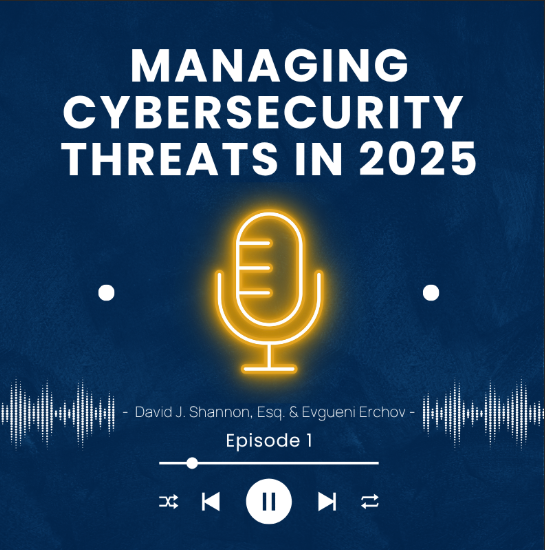
Managing Cybersecurity Threats in 2025 Episode 1
In this engaging episode of the PLUS Podcast, our host, David Shannon,…
Related Articles
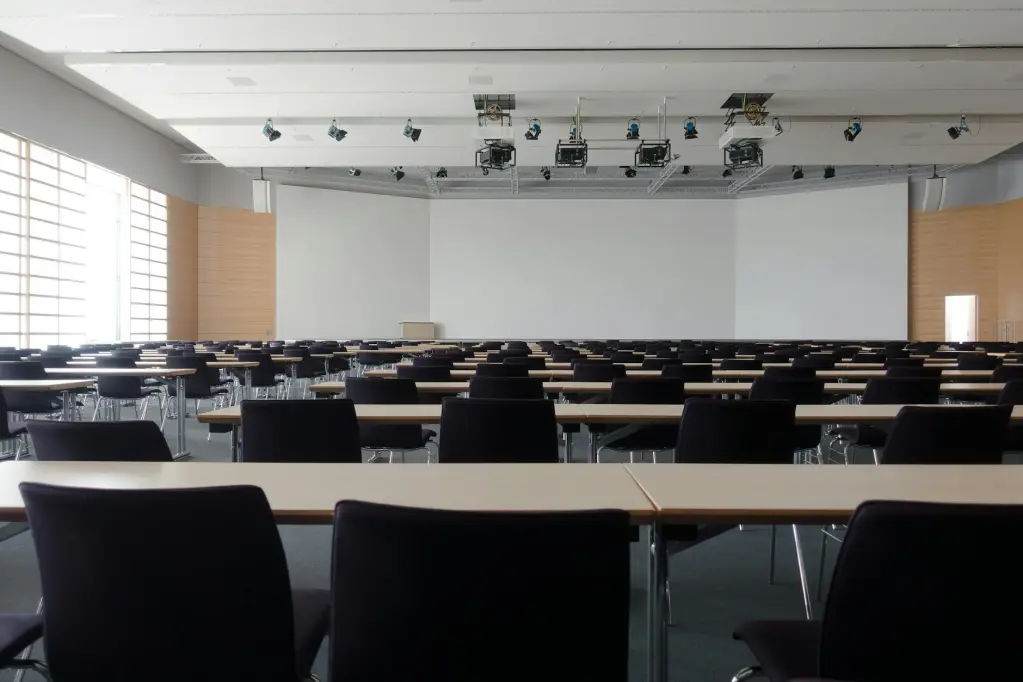
Cyber University 2025: Event Highlights & On‑Demand Access Available
PLUS hosted Cyber University on June 24th–26th, a comprehensive three-day program designed…
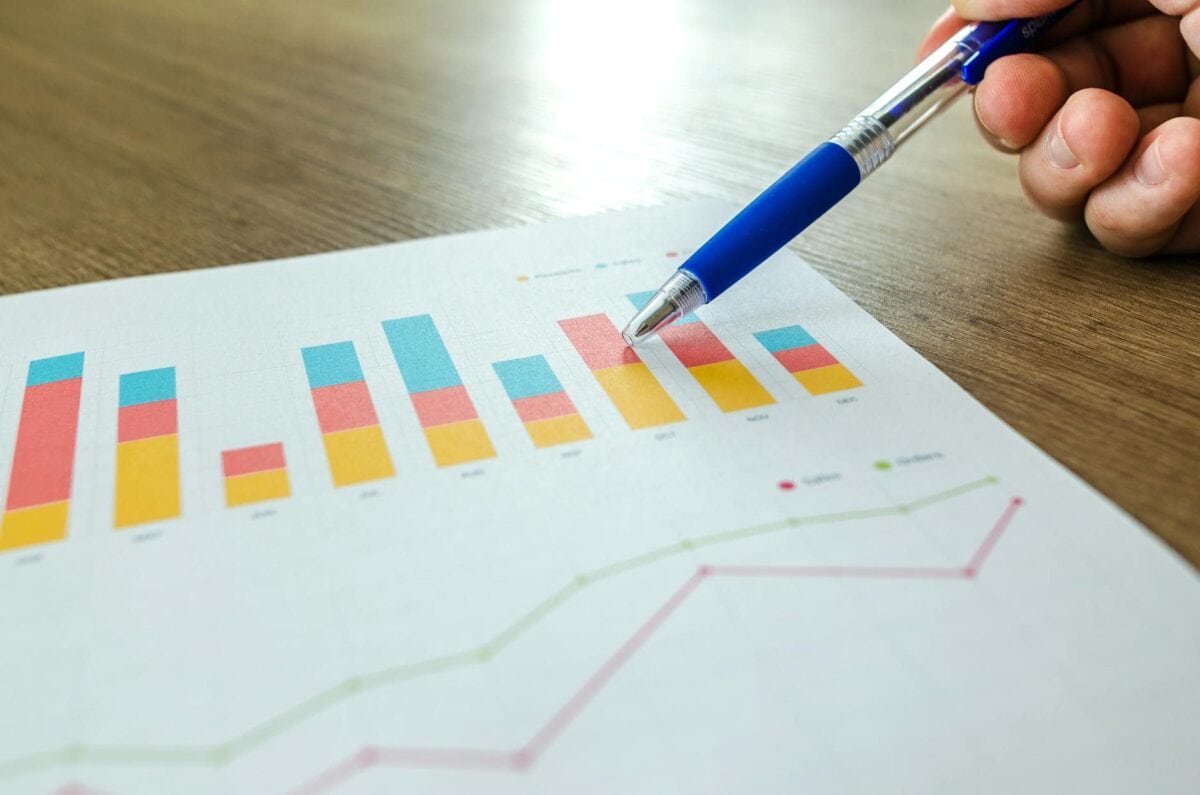
Professional Liability Market: Key Insights and Trends to Look Out For
Living in today’s progressively contentious and risk-sensitive environment, professional liability insurance takes…
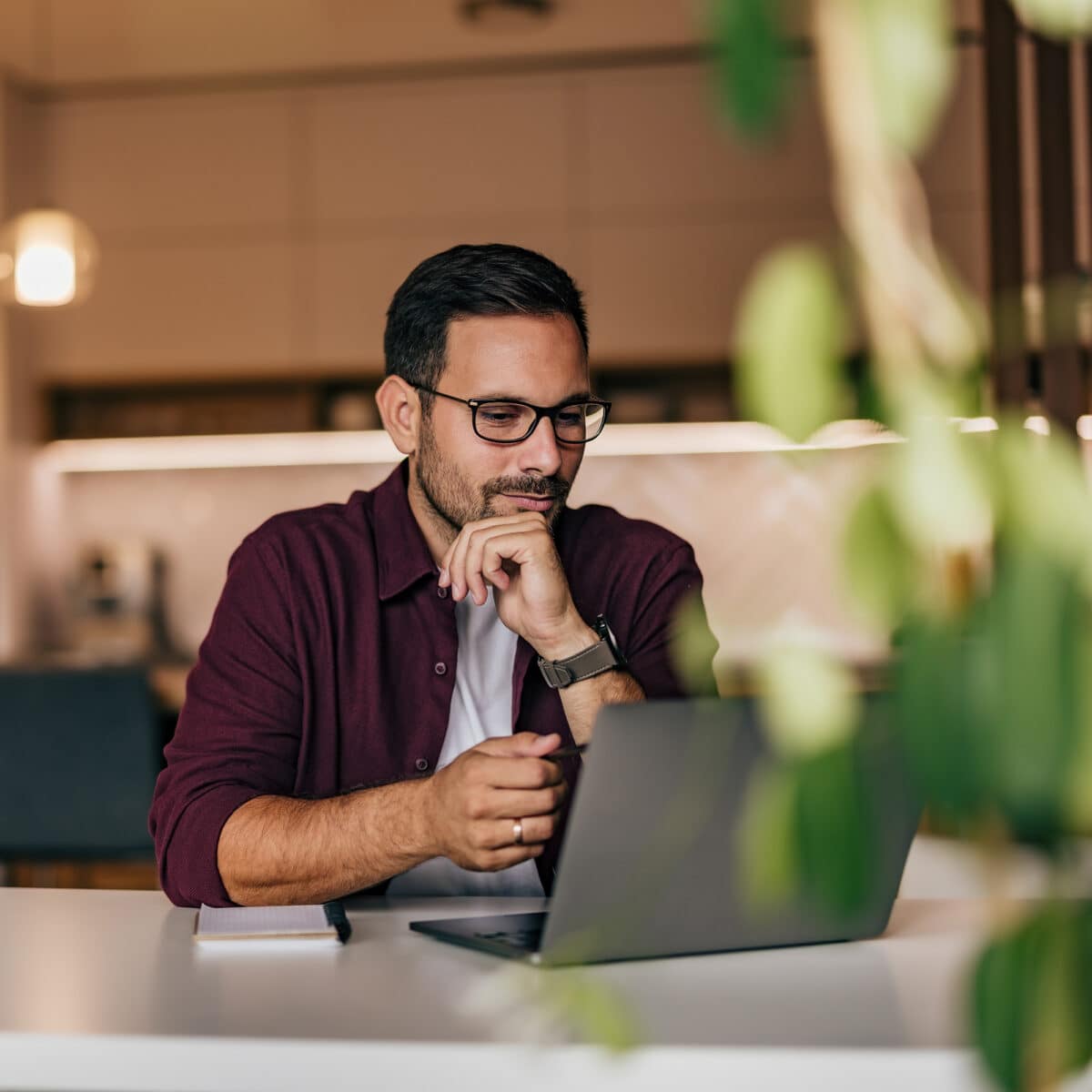
Ready to Expand Your Expertise in Cyber Insurance?
The 2025 Cyber University, taking place virtually June 24th–26th, is your ideal…